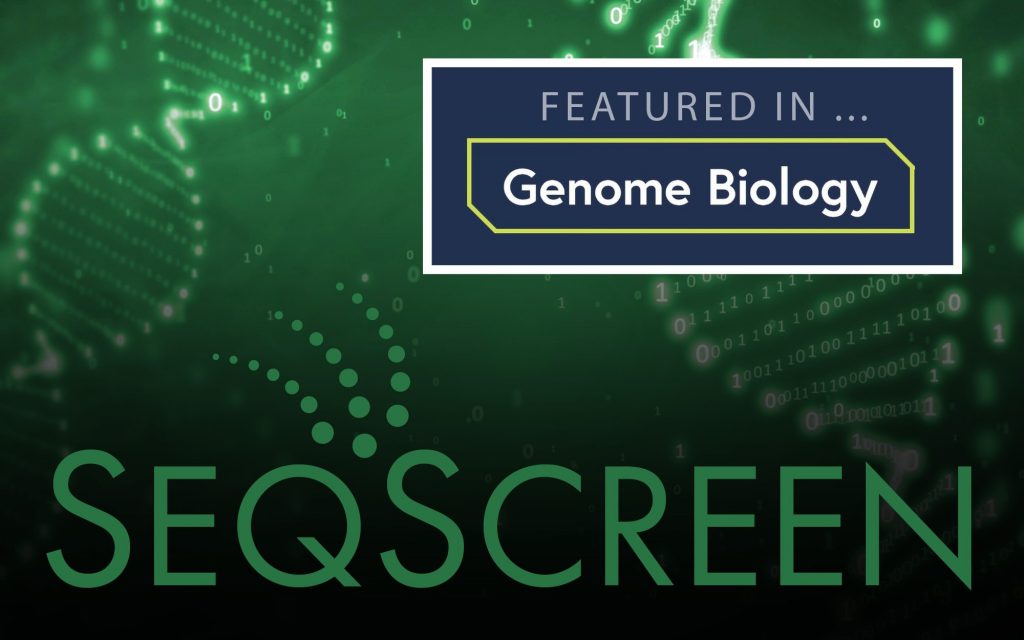
Abstract
The COVID-19 pandemic has emphasized the importance of accurate detection of known and emerging pathogens. However, robust characterization of pathogenic sequences remains an open challenge. To address this need we developed SeqScreen, which accurately characterizes short nucleotide sequences using taxonomic and functional labels and a customized set of curated Functions of Sequences of Concern (FunSoCs) specific to microbial pathogenesis. We show our ensemble machine learning model can label protein-coding sequences with FunSoCs with high recall and precision. SeqScreen is a step towards a novel paradigm of functionally informed synthetic DNA screening and pathogen characterization, available for download at www.gitlab.com/treangenlab/seqscreen.
Read the full paper here.
Authors
Advait Balajia, Bryce Killea, Anthony D. Kappellb, Gene D. Godboldc, Madeline Diepd, R. A. Leo Elwortha, Zhiqin Qiana, Dreycey Albina, Daniel J. Naskoe, Nidhi Shahe, Mihai Pope, Santiago Segarraf, Krista L. Ternusb & Todd J. Treangena
a Department of Computer Science, Rice University, Houston, TX, USA
b Signature Science, LLC, Austin, TX, USA
c Signature Science, LLC, Charlottesville, VA, USA
dFraunhofer USA Center Mid-Atlantic CMA, Riverdale, MD, USA
eDepartment of Computer Science, University of Maryland, College Park, MD, USA
fDepartment of Electrical and Computer Engineering, Rice University, Houston, TX, USA